Invited Speaker
TBA
TBA
Paper Submission Due:
Feb. 14, 2025
Author Notification:
Mar. 18, 2025
Registration Due:
Mar. 24, 2025
Camera Ready Due:
Mar. 24, 2025
Conference Date:
Apr. 23-25, 2025
LNEE submission
Paper Submission Due:
Feb. 14, 2025
Author Notification:
Mar. 18, 2025
Registration Due:
Apr. 15, 2025
Conference Date:
Apr. 23-25, 2025
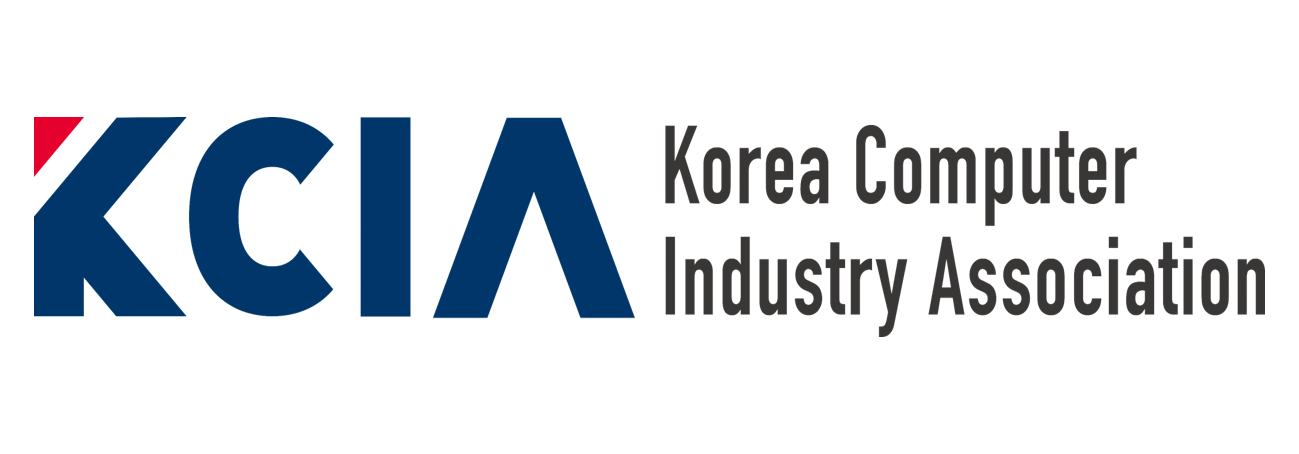